High quantity
Machine learning requires a lot of data. Often, the amount of real data we possess is not enough for machine learning. For complex applications and AI projects, collecting this large quantity of high-quality training data can be a challenge. Real training data also is collected in a linear way. Meanwhile, synthetic data can be generated in massive quantities, the main limits are the need for more processing power for even more examples. Along with the high quantity of data, there is also high-quality synthetic data. Rare events can occur more often in synthetic data to accurately train the AI model. Each object in a scene can also have a variety of annotations automatically generated which reduces efforts for data labelling.
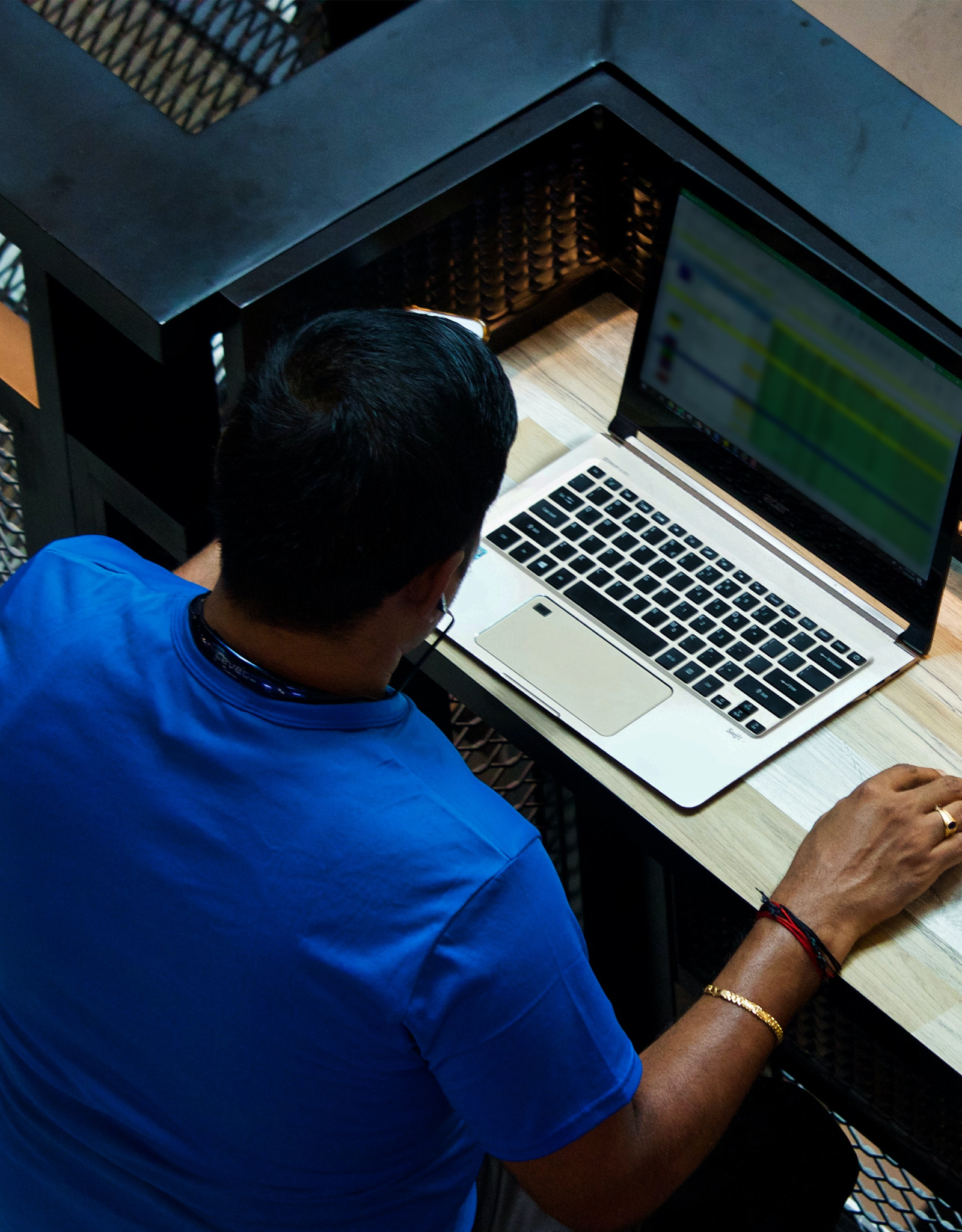